Computational empiricism : The reigning épistémè of the sciences
Philosophy World Democracy
What do mainstream scientists acknowledge as original scientific contributions, that is, what is the current épistémè in natural sciences?
Abstract
What do mainstream scientists acknowledge as original scientific contributions? In other words, what is the current épistémè in natural sciences? This essay attempts to characterize this épistémè as computational empiricism. Scientific works are primarily empirical, generating data and computational, to analyze them and reproduce them with models. This épistémè values primarily the investigation of specific phenomena and thus leads to the fragmentation of sciences. It also promotes attention-catching results showing limits of earlier theories. However, it consumes these theories since it does not renew them, leading more and more fields to be in a state of theory disruption.
Keywords: theory, statistical tests, empiricism, models, computation
Table of contents
Reading time: ~35 min
Computational empiricism : the reigning épistémè of the sciences
Abstract
What do mainstream scientists acknowledge as original scientific contributions? In other words, what is the current épistémè in natural sciences? This essay attempts to characterize this épistémè as computational empiricism. Scientific works are primarily empirical, generating data and computational, to analyze them and reproduce them with models. This épistémè values primarily the investigation of specific phenomena and thus leads to the fragmentation of sciences. It also promotes attention-catching results showing limits of earlier theories. However, it consumes these theories since it does not renew them, leading more and more fields to be in a state of theory disruption.
keywords: Philosophy, science
1 Introduction
Provided that, for better and worse, the historical model of modern sciences is classical mechanics, theories, and theorization used to have a central role in mainstream sciences. Then, the decline of theoretical thinking in sciences, the object of this special issue, becomes possible only once practitioners no longer feel the need for such work — or possibly when its possibility vanishes since the lack of possibility may very well translate into a lack of perceived need.
This decline requires a transformation in what is considered scientifically acceptable and what is acknowledged as scientific research. As such, we should ponder the nature of the dominant perspective of current sciences and the possibility that a new épistémè emerged. The justification of current practices lack sufficient elaboration and explicitness to shape a full-fledged doctrine and, a fortiori, a philosophy — though some of the components of these practices are highly refined. The most informal nature of the foundations of current practices seems necessary since it cannot withstand contradictions on intrinsic and extrinsic grounds — refutations have been numerous and compelling. Nevertheless, we hypothesize that it shapes both scientific institutions and everyday practice, sometimes by highly formalized procedures.
In a sense, several of its key texts are polemic, such as the one of Anderson (2008). However, we think that their aim is not to take genuine theoretical stands, but to shift Overton’s window, the range of ideas, and, here, of methods that mainstream practitioners consider sensible. We should acknowledge that this window has moved considerably. There are fields, such as molecular biology, where using artificial intelligence to generate hypotheses is received as a superb idea, and, by contrast, the very notion of theoretical or conceptual work has often become inconceivable.
To interpret this épistémè, let us not rush on the gaudy flags waved by some extreme authors and, instead, focus on the mainstream practice of sciences and its organization insofar as it has an epistemological dimension. To this end, we focus on some general but nevertheless precise characteristics of how current scientific work is structured intellectually.
2 The strange philosophical amalgam structuring scientific articles
In order to investigate the dominant épistémè in current sciences, let us start with the elementary unit of current scientific practice, namely the research article. We concur with Meadows when he states:
The construction of an acceptable research paper reflects the agreed view of the scientific community on what constitutes science. A study of the way papers are constructed at any point in time, therefore, tells us something about the scientific community at that time. (Meadows 1985)
In Foucauldian words, the structure of acceptable research articles provides evidence on the épistémè at a given time.
The prevailing norm for the structure of scientific articles is IMRaD; that is, Introduction, Methods, Results, and Discussion. This structure has been introduced in the 40s and 50s, depending on the disciplines. The American National Standards Institute (ANSI) formalized it as a standard, and its rule is still growing. It is enforced more or less rigorously in most scientific journals, especially in biology and medicine (Wu 2011).
The rationale of this norm is first to shape an article like an hourglass. The introduction goes from the general situation in a field to the specific question addressed by the article, the Methods and Results are narrow contributions, and the Discussion goes back from these results to their impact on the field of interest. In this sense, the main contribution of research articles is like a single brick added to the cathedral of scientific knowledge — especially when scientists aim for the minimal publishable unit, to further their bibliometric scores. However, since we consider that theoretical thinking requires reinterpreting empirical observations and theoretical accounts — not only in the field of interest but also in other relevant fields — this structure is deeply inimical to theoretical thinking. In other words, theorization is not about adding a brick in the edifice of a specific science; it involves rethinking its map or even its nature.
Let us proceed with the Discussion of the IMRaD structure. In 1964, P. Medawar, a Nobel prize winner, called this structure fraudulent, emphasizing the artificiality of the split between Results and Discussion:
The section called "results" consists of a stream of factual information in which it is considered extremely bad form to discuss the significance of the results you are getting. You have to pretend that your mind is, so to speak, a virgin receptacle, an empty vessel, for information which floods into it from the external world for no reason which you yourself have revealed. You reserve all appraisal of the scientific evidence until the "discussion" section, and in the Discussion, you adopt the ludicrous pretense of asking yourself if the information you have collected actually means anything. (Medawar 1964)
Medawar attributes this crooked structure to an inductive view of science, especially John Stuart Mill’s. Science would move from unbiased observations to knowledge. This perspective is philosophically dated; among many shortcomings, experimenting means bringing forth a specific situation in the world, motivated by a scientific stake and, therefore, endowed with interpretation and theoretical meaning. We add that, in theoretical thinking, a central question is the scientific interpretations of what it is that we can and should observe. For example, Einstein famously stated that:
Whether you can observe a thing or not depends on the theory which you use. It is the theory which decides what can be observed (Einstein, cited in Heisenberg 1971)
From this perspective, with IMRaD, the meaning of, say, an observed quantity is scattered between the Methods section that describes the procedure generating this quantity, the Results section that describes the outcome of this procedure, and the Discussion that interprets the results, notably in causal terms.
Even though Medawar does not discuss the Methods section much, we think its transformations in the last decades are worth discussing critically. The Methods section is often called Materials and Methods. Materials are the description of the concrete objects that scientists worked with, including the instruments of observation. Methods include sampling and transforming concrete objects, getting data from them, and analyzing these data.
Let us consider the statistical component of the Methods. For example, when observing different samples in an experiment, an argument is required to assess whether a result stems from chance or is evidence of causation. To this end, the primary method is the statistical test. Statistical tests are a kind of computational version of Popper’s falsification (Wilkinson 2013). First, tests require a null hypothesis; for example, treatment has no effects. Second, they require an alternative hypothesis, such as a decreased hospitalization rate in COVID-19 vaccines. Then, under the null hypothesis, the test estimates the probability of observing the experimental outcomes. If this probability is too low, the observations falsify the null hypothesis. Then, the latter is rejected in favor of the alternative.
There are many flaws with this method. For example, the typical threshold in biology is p=0.05, that is, one chance over twenty. However, this also means that it is sufficient to redo twenty times the same experiment or variations of it to have a good chance of a positive result — this is an explanation of why it is somewhat easy to provide empirical "evidence" of ESP (Extra-Sensory Perception) (McConway 2012).
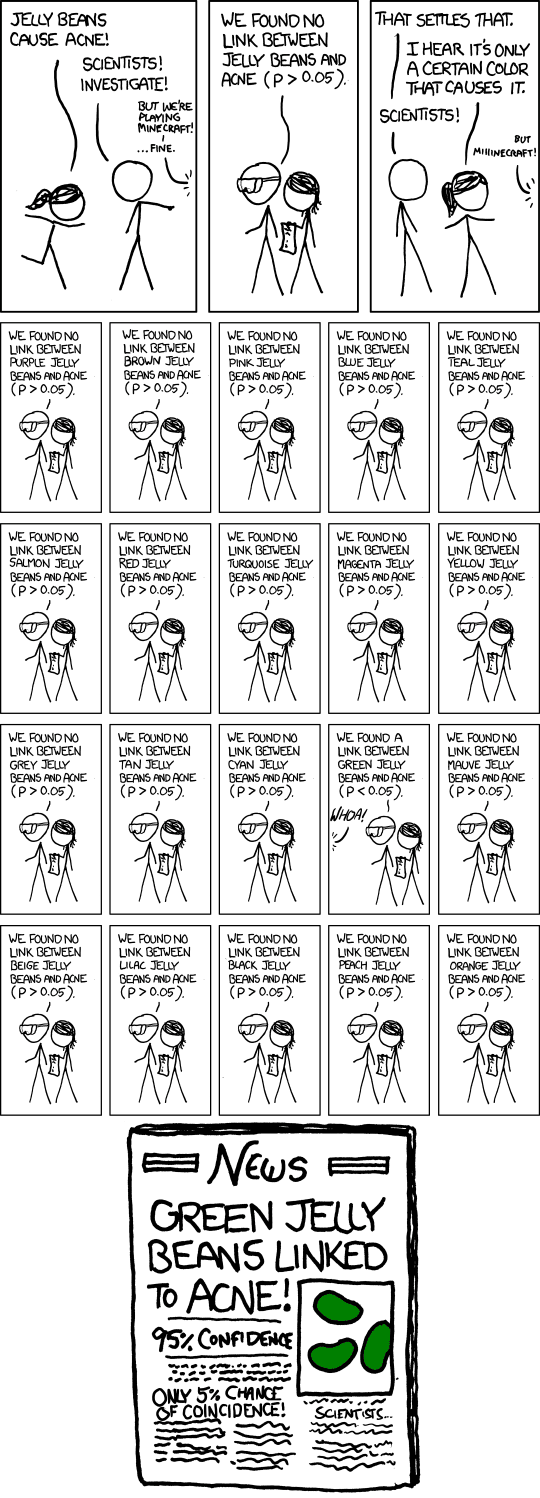
In this context, statistical tests appear as a general, almost automated way to assess whether an experiment yields “real” results or not. This automation, of course, is furthered by the use of user-friendly statistical software. The latter entails the usual dynamic of proletarianization, that is, the loss of knowledge following its transfer into the technological apparatus described by Marx and reworked by Bernard Stiegler (Stiegler 2018). In many cases, none of the authors of an article understand the concepts underlying these tests. As a result, tests have become rules of the experimentation and publication game and not an object of healthy controversy. Statisticians protested collectively against this situation in an unusual statement by the American Statistical Society (Wasserstein and Lazar 2016; Amrhein et al. 2019); however, this stance has no systemic consequences for now.
Since statistics require a population and are about collective properties, in this mainstream methodology of experimental science, case studies do not play any role and sometimes seem inconceivable to the practitioners. Nevertheless, among many other examples, it is still crucial in biology to define new species, in medicine to show that procedures like organ transplants are possible, or in astronomy to argue that an exoplanet exists in a specific system. This point brings another aspect of the dominant épistémè into light, namely the positivist influence. Indeed, the predominant aim is finding causal patterns such as "mechanisms" or mathematical relationships. By contrast, case studies provide a very different epistemological contribution; they show that something exists and a fortiori that something is possible — which may have profound practical and theoretical ramifications.
Overall, the disconnection between the Methods section and the critical examination in the Discussion is conducive to scientific writing and thinking protocolization. Experimental and analytic methods, including statistical ones, are described to be reproduced by other practitioners. In the context of the crisis in the reproducibility of experimental results (Baker 2016), this trend has gained momentum, emphasizing the transparency in the publication of the methods employed (Teytelman 2018). We have no qualms with an increase in transparency and in emphasizing reproducibility —especially if the same norm is applied to the scientific output of industry, for example, in the case of chemical toxicity investigations. However, we insist that protocolization can also be counterproductive since it downplays the work of objectivation, that is to say, the articulation between procedures and theoretical thinking. The choice of the quantities to observe, their robustness concerning details of the protocols are all questions that the IMRaD structure tends to marginalize. Neither the Methods nor the Results section accommodates naturally empirical or mathematical works aiming to justify the methods. Moreover, an underlying problem is widespread confusion between objectivity — an admittedly problematic notion — and automation. For example, the automatic analysis of biological images may depend on their orientation that stems from the arbitrary choice of the microscope user — it is then automatized but yields arbitrary results. Separating the methods from the Discussion contributes structurally to this confusion between objectivation and automation.
Incidentally, the IMRaD structure is highly prevalent in biology and medicine; however, it is not as strong in physics, where mathematical modeling plays a central role. A brief investigation shows that in multidisciplinary journals following IMRad or some variant, physicists tend to escape this structure, mainly by merging Results and Discussion or by transgressing the rationale of IMRaD sections shamelessly, often with the welcome complicity of editors and reviewers ... or by twisting their arms. We come back to the case of modeling below.
A key reason why this article structure dominates biomedicine is the massification and acceleration of scientific production. With a standard structure, hurried readers can find the same kind of information in the same place in all articles. In this sense, all articles have to follow the same overall rationale because scientists do not have the time to engage with specific ways to organize scientific rationality. The information paradigm is relevant to understand this situation. In information theory, the sender sends a message to the receiver; however, neither of them changes in this process. Articles following a standard structure — such as IMRaD — assume that the architecture of thinking can and should remain unchanged, and in this sense, these articles provide information about phenomena. Again, there is a gap with theoretical thinking since the latter aims precisely to change how we think about phenomena and address them scientifically.
Let us now put these elements together to develop a first description of the épistémè we are discussing. This épistémè builds on induction and is a kind of empiricism. At the same time, it typically uses a computational Popperian scheme to decide whether the results are genuine or the outcome of chance alone. The contradiction between the two philosophical stances is strong. However, it may escape many practitioners due to the protocolization or even the mechanization of scientific practices as typically described in the Methods sections. Moreover, once implemented in a computer, a statistical test is no longer primarily a scientific hypothesis to refute; instead, it becomes a concrete mechanical process to trigger. In a sense, in everyday biomedical practice, computers transformed statistical tests into an empirical practice, where the device (the computer) produces a result that can be faithfully published.
The IMRaD structure and the common use of statistics are not relevant to the complete scientific literature. Let us now discuss two other kinds of contributions: first, evidence-based medicine and its use of review articles, and then, mathematical modeling.
3 Evidence-based medicine and review articles
Evidence-based medicine is somewhat unique because it is genuinely a doctrine organizing medical knowledge — this statement does not imply that we concur with this doctrine. Prescriptions for original experimental research follow the IMRaD structure, and our Discussion above applies. Double-blind, randomized trials are the gold standard of the experiment, and statistical tests discriminate whether they provide conclusive evidence for or against the putative treatment. There are precise reasons for this method: in several cases, reasonable hypotheses on the benefit of drugs or procedures used to be broadly followed by medical care practitioners and were proven false by randomized trials.
However, this standard also means that the organization of medical knowledge does not accommodate theoretical considerations, and therefore, the latter provides a limited contribution to medical knowledge. Evidence-based medicine distrusts of theory may come from a confusion between theory and hypothesis. A theory provides a framework to understand phenomena; notably, it specifies what causality means in a field. For example, in classical mechanics, causes are forces, i.e., what pushes an object out of the state of inertia. In molecular biology, DNA plays the role of a prime mover, and effects trickle down from it. By contrast, hypotheses discussed in medicine posit that a specific process takes place and yields a given outcome. The theoretical issue with the latter is that, even though the putative process may indeed occur and the local hypothesis may be correct, other processes can be triggered by the treatment, some of which may be detrimental, leading to more risks than benefits. Incidentally, these other processes can also be therapeutically interesting; for example, Viagra resulted from investigating a drug against heart diseases.
Moreover, for methodological reasons, this doctrine implies that evidence only pertains to the effects on a given population (meaning here a collection of individuals on which the clinical trial was performed). A political shortcoming in these cases is that the population used is often rather specific; it typically corresponds to the North American or West European populations. Other populations may have frequent, relevant biological differences — not only for genetic reasons but also due to differences in their milieu and culture. Last, patient individuality and individuation are not entirely ignored by evidence-based medicine, but they are rather left entirely to practitioners’ experience: again, they cannot be the object of evidence for methodological reasons (Montévil In Press). Needless to say, this perspective is also a regression with respect to Canghuilhem’s critic of health as the statistical norm and his alternative concept of health as normativity (Canguilhem 1972)
A noteworthy aspect of evidence-based medicine is that it provides a global perspective on the organization of medical knowledge targeting the practical work of healthcare practitioners. Its founders considered the massification of publications mentioned above and the need for practitioners to acquire the most recent evidence relevant for the cases encountered. To this end, evidence-based medicine institutionalized review articles. These articles synthesize the results of primary research articles to conclude on the efficacy of one protocol or another so that practitioners, who have limited time to make decisions, do not have to read numerous articles. A recent, notable trend is to perform meta-analyses, that is to say, to put the results of different trials together in order to provide a statistical conclusion — again, statistical computations are the gold standard of evidence. Review articles also raise other considerations, sometimes even conceptual or epistemological. Nevertheless, they are no genuine substitute for dedicated theoretical research. The latter also synthesize a diversity of empirical work, but under the umbrella of a new way to consider the phenomena of interest in relation to other phenomena and theoretical perspectives.
The needs of medical practitioners also exist in fundamental research due to the massification and acceleration of scientific publications. Therefore, review articles are central in current sciences, and in a sense, are the locus of most synthetic works taking place in research. However, they are also in a very ambivalent position. First, journal editors typically commission reviews —and editors are not academics in many "top journals." Thus, the initiative to write and publish review articles does not come from authors and sometimes does not even come from research scientists.
Second, writing, publishing, and reading review articles carry a fundamental ambiguity that can be made explicit by distinguishing between analytic and synthetic judgments (or other concepts that may justify that a contribution is scientifically original). This question is raised provided that reviews do not contribute new empirical data and are not supposed to develop an entirely new perspective. Our aim, here, is not primarily to examine the nature of the reasoning taking place in these articles, like in the fundamental question of mathematics’ analytic or synthetic nature in Kant critic or the analytic stance of the Hilbert program. Instead, we aim to examine the current épistémè, and accordingly, we are interested in how the scientific community acknowledges review articles. For example, do scientists consider that review articles are primarily analytic recombinations of previously published results, or on the opposite, do they provide new insights?
Institutions and singularly scientific journals provide a very clear answer to this question. Review articles are typically published in contrast to original research and explicitly exclude them. This situation does not imply that original contributions do not take place in standard review articles, such as the critical Discussion of empirical results or hypotheses — editors typically require the review work to be critical. Now, a notable exception to the judgment on reviews is when statistical meta-analyses are performed. In the latter case, they may be considered original research. In other words, it seems that the computational nature of meta-analyses provides them with higher originality recognition than the critical arguments of other reviews.
We consider that theoretical works have a synthetic function. Review articles have largely taken over this function. They sometimes bring up theoretical considerations; nevertheless, they are not considered original research. The main exception is when original computations are performed in meta-analyses.
4 The case of mathematical modeling
Let us now discuss mathematical modeling in mainstream scientific practice. Mathematical modeling is often considered theoretical, and this is correct, of course, when theory is understood by contrast with empirical investigations. However, here, we sharply distinguish modeling from what we call theoretical work. Theory and models are distinct in fields such as physics or evolutionary biology. Moreover, they correspond to different research activities, playing different roles. Let us now discuss these points.
The notion of mathematical model carried significant epistemological weight in the 50s. For example, Hodgkin and Huxley described their mathematical work on neuron action potentials as a description and not a model (Hodgkin and Huxley 1952) — the vocabulary shifted, and it is now known as the Hodgkin Huxley model. Similarly, Turing contrasted his model of morphogenesis with the imitation of intelligence by computers (Turing 1950; Turing 1952). In current practice, the notion of a model is laxer than in the previous period. It encompasses computational models, that is to say, models whose only interpretable outcomes come from computer simulations.
Statistics aside, modeling is currently the most popular use of mathematics to understand natural phenomena. A central difference between models and theoretical thinking is that mathematical models are primarily local. They are concerned with a narrow, specific phenomenon, for example, the trajectories of the Earth and the Moon or the formation of action potentials by the combined action of several ionic channels in neurons.
Nevertheless, models can have a variety of epistemological roles concerning theories. For example, Turing’s model of morphogenesis can be interpreted as a falsification of the notion that biological development requires something like a computer program (Longo 2019). Another example is Max Planck’s discretization of energy — the idea that energy should not be seen as continuous but as small packets. This discretization was initially a modeling effort, aiming to understand the observations of light ray emission. Max Planck later stated:
[ It was] a purely formal assumption, and I really did not give it much thought except that no matter what the cost, I must bring about a positive result (Kragh 2000).
This daring modeling move was not theoretical by itself precisely because Max Planck did not, in his own terms, give it much thought. Nevertheless, this assumption was in profound contradiction with classical physics (classical mechanics and thermodynamics) because discreteness is not compatible with continuous deterministic change. It became the starting point of quantum mechanics, a revolutionary theoretical framework in physics that required to rethink observations, the nature of objects, determinism, and even logic. Let us also mention that mathematical contributions to theoretical thinking do not always go through models. A prominent example of this is the relationship between invariance and symmetry that Emmy Noether brought to light with her famous theorem. Beyond solving a pressing concern about energy conservation in general relativity, Noether’s theorem reinterpreted critical aspects of the structure of physics’ theories and became one of the most fundamental mathematical tools in contemporary theoretical physics (Bailly and Longo 2011).
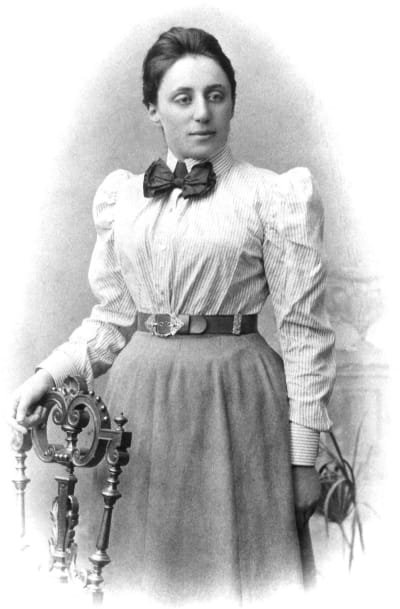
Now, the case of Max Planck’s discretization of energy shows that mathematical modeling requires a specific theoretical work about the integration in a broader framework to provide a genuine theoretical contribution and not just the opportunity for one. Most works on mathematical models do not contribute general theoretical considerations. Instead, they are more or less ad hoc accounts of a specific phenomenon, aiming to imitate several of its properties with precision. The contribution of the model is then narrow because it pertains only to a specific phenomenon. Thus, the contribution of these mathematical models and their publication structure follows the hourglass’s logic, as IMRaD articles, where texts go from broader considerations to a narrow contribution and finally back to a broader discussion. In this conception, like for empirical works, controversies are primarily local; they pertain to the elements and the specific formalism needed to account for the intended phenomenon.
In this sense, mathematical models are primarily local. Nevertheless, they may build on established theories, such as in many physics models. For example, models of the trajectories of the Earth and the Moon build on Galilean relativity, Newton’s universal gravitation, and Newtonian mechanics. The backbone of these principles is made explicit by numerous earlier theoretical discussions and results, such as Noether theorem. Thus, these models build on earlier work theorizing numerous empirical observations and mathematical and epistemological considerations. This situation explains why an elementary change in the mathematical structure of a model, such as Max Planck’s discretization, can be the starting point of a revolution once considered a theoretical move. Thus, theoretical work confers profound scientific meaning to the properties of models.
However, in situations where no theoretical framework pre-exists, the principles used to model a phenomenon are themselves local and typically ad hoc. In the absence of theoretical discussions, the meaning of models’ features remains shallow, and the modeling literature is rich in contradictions that are not elaborated upon and that accumulate, even in relatively narrow topics (Montévil et al. 2016). At the same time, the lack of interest in enriching models with theoretical meaning leads to conceiving mathematics as a tool, and the same tools tend to be used in all disciplines, a situation that the mathematician and epistemologist Nicolas Bouleau calls
To conclude, mathematical modeling is sometimes described as theoretical work; however, its local nature differs from working on theories. Current works emphasize the computational aspect of models, their ability to fit empirical data concerning the intended phenomenon and possibly make predictions. For these local developments, practitioners tend to think that the coherence and integration with other models and theoretical frameworks are not needed; however, this belief also implies that models’ features remain superficial, and, therefore, arbitrary.
5 Discussion
Let us conclude on the way we can characterize the épistémè that predominates in current sciences.
- Original scientific works are primarily empirical, generating data, and computational to analyze these data and reproduce them with models In empirical works, the overarching rationale is inductive, but they also require statistical computations that use Popper-inspired tests to discriminate whether results are significant. In computational models, the ability to fit empirical data is the central criterion by contrast with theoretical consistency or significance.
- Computations are ambivalent, especially since the invention of computers. Computers implement mathematical models, and at the same time, computations are processes that users can trigger without mathematical knowledge. We distinguish calculus from computations. The first corresponds to mathematical transformations that are theorized with mathematical structures and from which theoretically meaningful results may be pulled out, while the second corresponds to the mechanizable execution of digital operations.
- This épistémè requires the input of concrete objects, empirical or computational. By contrast, it does not value theoretical interpretation and reinterpretation.
- Critical synthetic works are performed primarily in review articles. They are not considered original research except when new computations are performed in meta-analyses.
- This épistémè primarily investigates specific phenomena and its contributions are supposed to be bricks contributing to the edifice of knowledge. In other words, it shares the cumulative view of positivism. By contrast, it marginalizes theoretical works that rethink how we understand phenomena by integrating a diversity of considerations.
On this basis, it seems reasonable to name this épistémè computational empiricism. Computational empiricism has a strong empiricist stance like logical empiricism; however, its stakes are different. Logical empiricism posits that science is about analytical (logical) or empirically verified truth. By contrast, computational empiricism focuses on empirical data and mechanized computations. It cares for numerical questions (like statistics); however, it does not attend its logoi significantly, by analytical means or otherwise.
Computational empiricism is an industrialization of research activity, a paradoxical notion considering that research is about bringing new, singular insights. To accommodate this tension, the original works it acknowledges are local; by contrast with the theoretical works that have precisely a synthetic function. Accordingly, it leads to the fragmentation of research work and scientific knowledge, and it goes with an institutional fragmentation, where different laboratories address similar questions with similar means on slightly different objects. The underlying government of science expects that interesting results will emerge by probing the world in many different places without attending to scientific reflexivity. In some cases, like molecular biology or the human brain project, the underlying idea is to decompose complex objects (living beings or the brain respectively), so that each laboratory studies a few aspects of it (a molecule, a pathway or a neuronal circuit) under the assumption that the result of these studies could somehow be combined. This organization goes with a cumulative view of science, where productivism is a natural aim. In this sense, computational empiricism aims primarily to extract patterns from nature, considering that some of them may be usable. Politically, it means that theoretical controversies no longer can set a field ablaze, leading to the bifurcation of its perspective on their phenomena of interest. Thus, partition yields academic peace, even though it might very well be the peace of cemeteries.
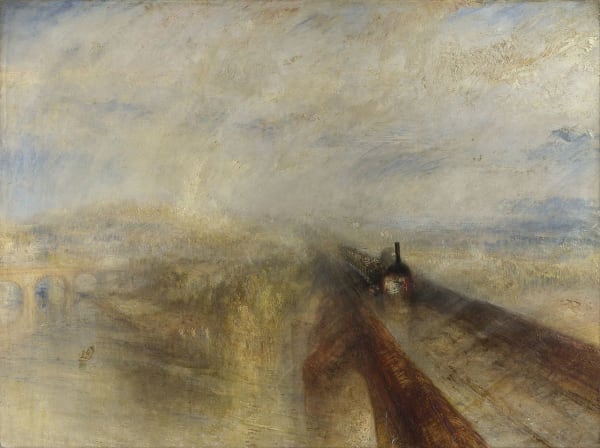
Computational empiricism logically values technological innovations highly, whether experimental or computational — especially when they have transversal applications. Similarly, the deployment of recent innovation on new objects are natural activities in this épistémè. In a somewhat perverse way, it also promotes empirical results that destabilize former theoretical frameworks because they catch attention. However, it does not promote rethinking these frameworks, leading to what may be called a theory disruption that is more or less advanced depending on the fields and is a component of the disruption diagnosed by Bernard Stiegler (Stiegler 2019).
Computational empiricism has substantial inconsistencies in its current form. In empirical articles, one of them is the need to formalize statistical hypotheses for statistical tests. Indeed, a Popper-inspired scheme does not integrate well with an entirely inductive rationale. Opportunely, deep learning provides methods to find patterns in big data. It is then not surprising that a strong current pushes forward the idea to generate hypotheses by artificial intelligence methods (Kitano 2016; Peterson et al. 2021). Kitano notably puts forward the aim for artificial intelligence to provide Nobel prize level contributions (Kitano 2021). More concretely, Peterson et al. (2021) use deep learning to generate "theories" of human decision making; however, we can also note that this method requires theoretical constraints to produce interpretable "theories". Computational empiricism is not a consistent doctrine, and the repressed need for theoretical insights always makes a return.
Peterson et al. (2021) make very explicit their use of prior theoretical considerations; however, others argue differently on far more informal grounds (Anderson 2008). Computers do not only provide automation of computations and derived tasks such as classification or optimization. They are also an efficient artificial metis as proposed and made explicit by Turing in the imitation game (Turing 1950). As such, they can be used to generate epistemological illusions, notably the illusion of scientific research without theorization. However, such an illusion would not have taken hold without an épistémè preparing the minds for it.
Of course, counter forces are calling for theoretical work in various fields and with diverse epistemological and theoretical stances (Soto et al. 2016; Muthukrishna and Henrich 2019; O’Connor et al. 2019). At the institutional level, the call for interdisciplinarity may be a clumsy method to promote synthetic theoretical works, even though interdisciplinarity can also regress to the division of labor that finds its home in computational empiricism. An opposite perspective would be to distinguish, for example, the principles of construction from the principles of proof in scientific knowledge (Bailly and Longo 2011). Computational empiricism only values the principles of proof (empirical and computational). An alternative should recognize the theoretical elaboration of knowledge again as critical for science.
References
- Amrhein, V., S. Greenland, B. McShane, and 800 signatories (2019). “Scientists rise up against statistical significance”. In: Nature 567, pp. 305–307. doi: 10.1038/d41586- 019-00857-9.
- Anderson, C. (2008). “The end of theory: The data deluge makes the scientific method obsolete”. In: Wired magazine 16.7, pp. 16–07.
- Bailly, F. and G. Longo (2011). Mathematics and the natural sciences; The Physical Singularity of Life. London: Imperial College Press. doi: 10.1142/p774.
- Baker, M. (2016). “1,500 scientists lift the lid on reproducibility”. In: Nature 533, pp. 452–454. doi: 10.1038/533452a.
- Bouleau, N. (2021). Ce que Nature sait: La révolution combinatoire de la biologie et ses dangers. Presses Universitaires de France.
- Canguilhem, G. (1972). Le normal et le pathologique. Paris: Presses Universitaires de France.
- Heisenberg, W. (1971). Physics and Beyond. New York: Harper.
- Hodgkin, A. L. and A. F. Huxley (1952). “A quantitative description of membrane current and its application to conduction and excitation in nerve”. In: The Journal of physiology 117.4, pp. 500–544.
- Kitano, H. (Apr. 2016). “Artificial Intelligence to Win the Nobel Prize and Beyond: Creating the Engine for Scientific Discovery”. In: AI Magazine 37.1, pp. 39–49. doi: 10.1609/aimag.v37i1.2642.
- Kitano, H. (June 2021). “Nobel Turing Challenge: creating the engine for scientific discovery”. In: npj Systems Biology and Applications 7.1, p. 29. issn: 2056-7189. doi: 10.1038/s41540-021-00189-3.
- Kragh, H. (2000). “Max Planck: the reluctant revolutionary”. In: Physics World.
- Longo, G. (2019). “Letter to Turing”. In: Theory, Culture & Society 36.6, pp. 73–94. doi: 10.1177/0263276418769733.
- McConway, K. (2012). “Understanding uncertainty: ESP and the significance of significance”. In: Plus magazine.
- Meadows, A. (1985). “The scientific paper as an archaeological artefact”. In: Journal of Information Science 11.1, pp. 27–30. doi: 10.1177/016555158501100104.
- Medawar, P. (Aug. 1964). “Is the Scientific Paper Fraudulent?” In: The Saturday Review.
- Montévil, M. (In Press). “Conceptual and theoretical specifications for accuracy in medicine”. In: Personalized Medicine in the Making: Philosophical Perspectives from Biology to Healthcare. Ed. by C. Beneduce and M. Bertolaso. Human Perspectives in Health Sciences and Technology. Springer. isbn: 9783030748036.
- Montévil, M., L. Speroni, C. Sonnenschein, and A. M. Soto (Aug. 2016). “Modeling mammary organogenesis from biological first principles: Cells and their physical constraints”. In: Progress in Biophysics and Molecular Biology 122.1, pp. 58–69. issn: 0079-6107. doi: 10.1016/j.pbiomolbio.2016.08.004.
- Muthukrishna, M. and J. Henrich (Mar. 2019). “A problem in theory”. In: Nature Human Behaviour 3.3, pp. 221–229. issn: 2397-3374. doi: 10.1038/s41562-018-0522-1.
- O’Connor, M. I., M. W. Pennell, F. Altermatt, B. Matthews, C. J. Melián, and A. Gonzalez (2019). “Principles of Ecology Revisited: Integrating Information and Ecological Theories for a More Unified Science”. In: Frontiers in Ecology and Evolution 7, p. 219. issn: 2296-701X. doi: 10.3389/fevo.2019.00219.
- Peterson, J. C., D. D. Bourgin, M. Agrawal, D. Reichman, and T. L. Griffiths (2021). “Using large-scale experiments and machine learning to discover theories of human decision-making”. In: Science 372.6547, pp. 1209–1214. issn: 0036-8075. doi: 10.1126/ science.abe2629.
- Soto, A. M., G. Longo, D. Noble, N. Perret, M. Montévil, C. Sonnenschein, M. Mossio, A. Pocheville, P.-A. Miquel, and S.-Y. Hwang (Oct. 2016). “From the century of the genome to the century of the organism: New theoretical approaches”. In: Progress in Biophysics and Molecular Biology, Special issue 122.1, pp. 1–82.
- Stiegler, B. (2018). Automatic society: The future of work. John Wiley & Sons.
- Stiegler, B. (2019). The Age of Disruption: Technology and Madness in Computational Capitalism. Cambridge, UK: Polity Press. isbn: 9781509529278.
- Teytelman, L. (2018). “No more excuses for non-reproducible methods.” In: Nature 560, p. 411. doi: 10.1038/d41586-018-06008-w.
- Turing, A. M. (1950). “Computing machinery and intelligence”. In: Mind 59.236, pp. 433–460.
- Turing, A. M. (1952). “The Chemical Basis of Morphogenesis”. In: Philosophical Transactions of the Royal Society of London. Series B, Biological Sciences 237.641, pp. 37–72. doi: 10.1098/rstb.1952.0012.
- Wasserstein, R. L. and N. A. Lazar (2016). “The ASA Statement on p-Values: Context, Process, and Purpose”. In: The American Statistician 70.2, pp. 129–133. doi: 10.1080/ 00031305.2016.1154108.
- Wilkinson, M. (May 2013). “Testing the null hypothesis: The forgotten legacy of Karl Popper?” In: Journal of Sports Sciences 31.9, pp. 919–920. doi: 10.1080/02640414. 2012.753636.
- Wu, J. (Dec. 2011). “Improving the writing of research papers: IMRAD and beyond”. In: Landscape Ecology 26.10, pp. 1345–1349. issn: 1572-9761. doi: 10.1007/s10980-011- 9674-3.