Quantitative analysis of endocrine disruption by ketoconazole and diethylstilbestrol in rat mammary gland development
Reproductive Toxicology
Below is a podcast and transcript of the interview concerning the 1st chapter of the book Bifurquer
Abstract
Endocrine disruptors alter mammary gland development, impair the ability to nourish offspring, and increase the cancer risk in animal models. Epidemiological studies reveal trends towards early mammary development, nursing problems, and breast cancer in younger women. Morphological changes in mouse postnatal mammary gland development are considered sensitive markers of endocrine disruption. While the mouse mammary gland is easily amenable to morphometric measurements from the fetal stage to full maturity, the rat mammary gland grows more conspicuously into the third dimension, hindering conventional morphometric analysis. However, since rats are more commonly used in international toxicological reproductive studies, it would be beneficial to include mammary gland whole-mount analysis in these studies. Using our quantitative software to perform computer-driven analysis of the rat mammary epithelium we examined the effects of gestational and postnatal exposure to ketoconazole, an antifungal medication that affects steroidogenesis, and to the estrogen diethylstilbestrol in the mammary glands of 6- and 22-day-old females. Both treatments produced effects at both ages; the epithelium was smaller and less complex in exposed animals compared to controls. Global analysis with the permutation test showed that morphological evaluation of the PND22 mammary gland is sensitive to endocrine disruption and possibly non-monotonic. In addition to revealing that ketoconazole altered the mammary gland structure, these results suggest that for future toxicology studies, day 22 (at weaning) is more suitable than day 6 because it showed significant measurements and trends. If the collection of mammary glands is added to existing international test methods, PND22 could be a relevant time point.
Keywords: Ketoconazole, Diethylstilbestrol, endocrine disruptors, perinatal exposure, mammary gland whole mount
Table of contents
Reading time: ~49 min
- 1. Introduction
- 2. Materials and methods
- 2.1. Chemicals
- 2.2. Animals and dosing
- 2.3. Tissue Harvesting and Processing
- 2.4. Image Capture
- 2.5. Identification of the epithelial tissue
- 2.6. Identification of epithelial structures and extraction of quantitative epithelial morphological features
- 2.7. Statistical analysis of quantitative epithelial morphological features
- 3. Results
- 4. Discussion
- 5. Conclusions
- Appendix A. Supplementary material
- References
Quantitative analysis of endocrine disruption by ketoconazole and diethylstilbestrol in rat mammary gland development
Abstract
Endocrine disruptors alter mammary gland development, impair the ability to nourish offspring, and increase the cancer risk in animal models. Epidemiological studies reveal trends towards early mammary development, nursing problems, and breast cancer in younger women. Morphological changes in mouse postnatal mammary gland development are considered sensitive markers of endocrine disruption. While the mouse mammary gland is easily amenable to morphometric measurements from the fetal stage to full maturity, the rat mammary gland grows more conspicuously into the third dimension, hindering conventional morphometric analysis. However, since rats are more commonly used in international toxicological reproductive studies, it would be beneficial to include mammary gland whole-mount analysis in these studies. Using our quantitative software to perform computer-driven analysis of the rat mammary epithelium we examined the effects of gestational and postnatal exposure to ketoconazole, an antifungal medication that affects steroidogenesis, and to the estrogen diethylstilbestrol in the mammary glands of 6- and 22-day-old females. Both treatments produced effects at both ages; the epithelium was smaller and less complex in exposed animals compared to controls. Global analysis with the permutation test showed that morphological evaluation of the PND22 mammary gland is sensitive to endocrine disruption and possibly non-monotonic. In addition to revealing that ketoconazole altered the mammary gland structure, these results suggest that for future toxicology studies, day 22 (at weaning) is more suitable than day 6 because it showed significant measurements and trends. If the collection of mammary glands is added to existing international test methods, PND22 could be a relevant time point.
Abbreviations
1. Introduction
Endocrine- disrupting chemicals have been shown to alter mammary gland (MG) development, impair the ability to nourish offspring via lactation, increase mammary tissue density, and increase the propensity for developing cancer in animal models [23] . These results are consistent with epidemiological studies revealing trends toward early mammary development, nursing problems, and breast cancer in younger women [12] . Morphological changes in early mammary gland development are considered sensitive markers of endocrine disruption in rodents [30] , [4] . This is evaluated by image analysis of mammary gland whole mounts. Additionally, it has been known for almost 40 years that the mouse neonatal mammary gland is significantly more sensitive to estrogens than the uterus and the vagina [2] , a fact consistently observed in multiple studies, including those obtained after fetal exposures [17] , [18] , [30] , [32] , [34] , [36] . Thus, it is important to examine the potential of the rodent mammary gland as a target for disruption of female reproduction, given that several of the currently used tests and endpoints are quite insensitive to these chemicals [11] , [19] . It should be noted that the mammary gland is not only sensitive to estrogens, progesterone and prolactin but also to additional hormonal stimuli including vitamin D, androgens, thyroid hormones, etc. [24] , [37] , [7] , [8] . Moreover, the mammary gland responds to natural estrogens [35] and xenoestrogens [19] in a non-monotonic fashion. This fact further complicates the selection of appropriate methodologies for assessing toxicological effects, as a single high dose will not suffice if the most striking effects are found at low or intermediate doses [9] , [19] .
The rat is the preferred model for the study of female reproduction and, thus, for the internationally applied toxicological test methods involving reproductive endpoints. The rat is also considered a good model for the study of mammary gland carcinogenesis, particularly for those that are hormone-sensitive [1] , [21] , [22] . In order to include the mammary gland as an endpoint in these toxicological studies, methodologies need to be adjusted to work in rat tissue. One reason for this is that while the mouse mammary gland ductal tree is easy to analyze by standard morphometric methods, the rat mammary gland grows conspicuously into the third dimension, hindering the application of conventional morphometrics ( Fig. 1 ).
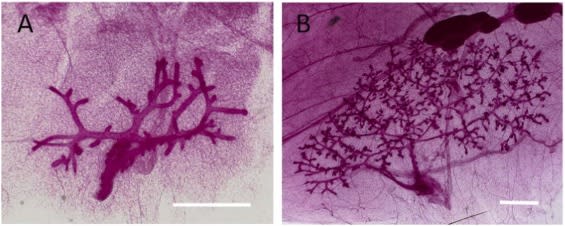
Fig. 1. Comparison of mouse (A) and rat (B) mammary glands at PND21–22. Scale bar: 1 mm.
To overcome this limitation, a new quantitative semi-automatic methodology was developed [19] . This method is used to obtain truly quantitative data, which is expected to validate the semiquantitative methods that are already available. Both methods have the advantage of providing a global evaluation of effects, rather than a single endpoint. In order to evaluate the morphological changes in the mammary gland, the shape of the entire gland is assessed, as well as individual characteristics of the epithelial structures. One way to accomplish this is by using the imaging processing program Image J ( https://imagej.nih.gov , and https://en.wikipedia.org/wiki/ImageJ ) using various existing plugins and writing custom scripts, changes in morphometry can be evaluated. (For a more detailed explanation of the plugins and custom scripts used, see Section 2.6 and supplemental information ). A methodology that results in over a hundred measurements per rat in an experiment where the animal number per group is one order of magnitude lower requires specific statistics, particularly when a substantial number of the variables that describe the mammary gland are not independent, and it is necessary to assess dose-response. Therefore, the statistical analysis has to assess the results globally; to this end, we use the permutation test, which is a robust method commonly used in other fields (the rationale for this approach is developed in the methods section).
The aim of the present study was two-fold, to assess the effect of ketoconazole in the mammary gland of rodents as clinical reports showed an association with gynecomastia in men treated with this fungicide [3] , as well as to assess the sensitivity of the rat mammary gland to proven model endocrine disruptors and to compare it with the traditional reproductive endpoints measured in the same animals. In this study, two time points were assessed, postnatal day (PND) 6 and PND22. The two ages allow for analysis before and after the ovaries begin secreting hormones. Additionally, the quantitative automatic methodology developed by Montévil, Acevedo et al. [19] was expanded and used to analyze the mammary glands of rats exposed to either one of two endocrine disruptors known to produce effects in humans, the synthetic estrogen diethylstilbestrol (DES) and the fungicide Ketoconazole (KETO) from gestational day (GD) 7 until PND22. While DES is a full estrogen agonist that acts via the nuclear estrogen receptors alpha and beta, KETO alters steroid hormone synthesis in humans and rodents by inhibiting various cytochrome P450 enzymes of the steroidogenesis pathway, interfering with both androgen and estrogen synthesis [11] , [13] , [20] . In spite of evidence that at high doses, KETO could inhibit aromatase and thus reduce estrogen production, men treated with KETO often experience gynecomastia [3] . This paradoxical result may be due to the inhibition of the hydroxylation (phase I) of estradiol and of its conjugation (phase II), thus decreasing the excretion of estradiol [27] , [28] .
This study was part of the FREIA project, which aimed to generate a better understanding of how endocrine disrupting chemicals (EDCs) impact female reproductive health and use this information to provide improved test methods to guide chemical regulation and improve the reproductive health of women [4] .
These experiments revealed that KETO alters the organization of the mammary gland epithelium. Additionally, by using the same set of animals to examine the mammary gland and the reproductive tract [11] we have found that the rat mammary gland is a more sensitive target than the traditional female reproductive endpoints, as was first reported by Bern et al., four decades ago in mice [2] .
2. Materials and methods
2.1. Chemicals
Ketoconazole (KETO, purity 98 %; CAS no. 65277–42–1) was obtained from BOC Sciences Inc. USA, and diethylstilbestrol (DES, purity ˃ 99 % CAS no. 56–53–1) and corn oil (used as vehicle and control, Cat. C8267–2.5 L) was obtained from Sigma-Aldrich. Dosing solutions were stored in the dark in glass bottles at room temperature and stirred continuously during the dosing period.
2.2. Animals and dosing
Animals for this study were generated for the in vivo study previously described in Johansson et al. [11] . The animal study was reviewed and approved by Danish Animal Experiments Inspectorate; authorization number 2015–15–0201–00553. All methods were performed and overseen by the in-house Animal Welfare Committee at DTU. Briefly, pregnant Sprague-Dawley rats (Crl:CD(SD)) were obtained (Charles River Laboratories, Sulzfeld, Germany) on GD3 with the day following overnight mating designated as GD1. Animals were fed a standard soy- and alfalfa-free diet based on Altromin 1314 (Altromin GmbH, Germany) and provided with tap water ad libitum in Bisphenol-A free bottles (Polysulfone 700 ml, Tecniplast, Italy). Food samples were tested for estrogenic activity in the E-SCREEN assay [31] and were below 5 pmol/g, a negligible amount. Rats were exposed orally via gavage from GD7 until PND22 to 0.003; 0.006; and 0.012 mg/kg bw/day DES and 3; 6; or 12 mg/kg bw/day KETO.
2.3. Tissue Harvesting and Processing
On PND6 and PND22, mammary glands (4th pair) were harvested and processed for carmine-stained whole mount analysis as described previously [16] . The glands were then assessed with blinded treatment groups, using the method in Montévil et al. [19] and described below.
2.4. Image Capture
Images of the PND6 and PND22 whole-mounted mammary glands were captured with a Zeiss LSM800 confocal microscope and reconstructed to generate a 3-D image of the whole gland. This was possible due to the auto-fluorescence of the carmine stain. The images were captured on a grid with overlapping stacks. Due to the differing sizes of the glands at the different ages collected, images were captured with a resolution of 1.25 µm for the PND6 and 2.5 µm for the PND22. Tiles were stitched together using the Zeiss software associated with the LSM800. Sample numbers of images collected were n = 10 per group with one exception: at PND6 for the KETO 3 mg dose, n = 11.
2.5. Identification of the epithelial tissue
Identification of the epithelium is done by segmentation, which separates a region of interest, in this case, the epithelium, from the stroma with its blood and lymph vessels using a custom automatic method [19] . For the current work, this method was modified due to the more complex periductal structure of the PND6 stroma when compared with PND22 glands. This problem required the development of a protocol using machine learning.
2.5.1. Preprocessing
The decreased brightness of structures deeper in the tissue was corrected based on the structures described above.
2.5.2. Machine learning
The Weka segmentation plugin of Image J ( https://imagej.net/plugins/tws/ ), was used to classify pixels of the images. Two classifiers were trained to this end on 2D slices of the image stacks. For better results, 9 categories were used, with two parts of the epithelium (main ducts and buds) and the others corresponding roughly to different features of the stroma (adipocytes, blood vessels, etc.). A support vector classifier and the fast random forest classifier of the Weka plugin were trained, and the resulting classifier was applied to the stacks, slice by slice ( Fig. 2 ).
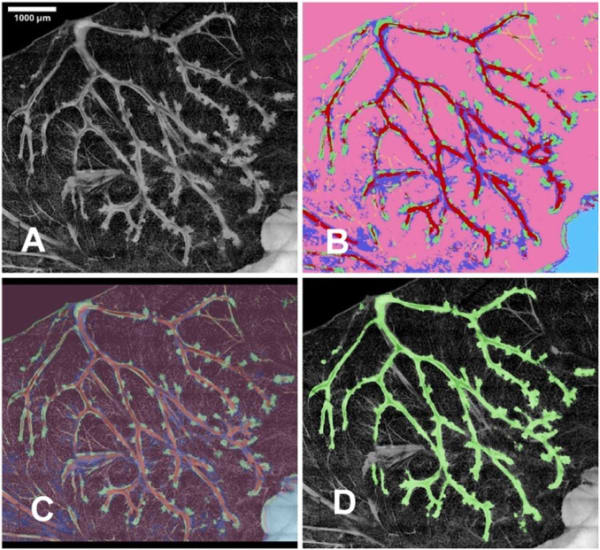
Fig. 2 . Classification of pixels by machine learning and the resulting segmentation for a 2D slice of a PND6 mammary gland 3D stack. Panel A: output of the confocal microscope after stitching. Panel B : Classification of the pixels by machine learning. Red pixels denote the principal epithelium (ducts) and green pixels denote the buds. These two colors correspond to the structure analyzed with the aim of maintaining the segmentation. The other colors correspond to different features of the stroma. The latter do not correspond to significant biological categories for this analysis since the aim was primarily to discard the stroma. Panel C : superposition of the upper left and right images to show the correspondence between classification of pixels and the original image. Panel D : segmentation result after the complete segmentation process.
2.5.3. Criterion for segmentation
A weighted mean between the result of the two classifiers and the intensity of the preprocessed image was used as the basis to identify the epithelium.
2.5.4. Semi-automatic segmentation
Segmentation identifies the largest component above a fixed threshold, fills holes in the structure (miscategorized lumen), and removes small artifacts. If the wrong structures are kept (for example, lymph vessels) or correct structures are missing (for example, due to cuts in the sample), then an additive and a subtractive stack are edited by the user. This will then be applied to the image used for this step, and this step will be iterated until the results are satisfactory ( Fig. 2 , Fig. 3 ). The result of segmentation is a 3D reconstruction of the epithelium.
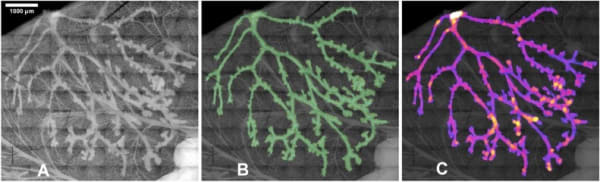
Fig. 3 . Projection (brighter pixel) of all slices of a PND6 MG stack. Panel A : Original image after stitching, Panel B: Green overlay of the epithelium after segmentation, Panel C: Analysis of the local thickness of the epithelium, warmer colors correspond to thicker parts of the epithelium in 3D.
2.6. Identification of epithelial structures and extraction of quantitative epithelial morphological features
Once segmentation was achieved, this representation of the epithelium was used to extract multiple quantitative morphological features. ImageJ, which analyzes particles, was used for initial basic measurements. The existing plugins to ImageJ were used: MorphoLibJ, which is a collection of mathematical morphology methods, analyses 2D shapes [15] , the plugin 3D shape [29] , and the BoneJ plugins (see supplemental information for more details). The latter included an evaluation of the local thickness ( Fig. 3 C). Examples of some assessed quantities are the aspect ratio (length/width), the epithelial area, and the fractal dimension of the epithelium in 2D (the projection of its 3D image). The same kind of global analysis was also performed in 3D and included an evaluation of the surface of the epithelium, its volume, and its 3D fractal dimension (based on the box-counting method). The skeletonized epithelium was analyzed by generic methods (counting the number of branches, average branch length, etc.). This analysis was performed both with and without terminal branches (pruning) since some of the terminal branches may not correspond to actual epithelial structures but may be artifacts from the skeletonization process. The number of parts categorized as buds by machine learning in the segmentation step and total bud volume were also counted. It should be noted that these structures are identified as buds by the software but may include very short branches.
We developed an approach that reconstructs the epithelial tree using a custom mammary gland analysis plugin [19] . This plugin uses the skeleton generated for previous endpoints and adds a manual selection of the starting point of the gland (the point of attachment to the skin). The plugin then reconstructs the mammary tree with the main duct as the root. This reconstruction was used as the basis for evaluating various properties. When quantities are defined per branch, the average of all branches is reported. To filter biologically relevant shapes, two versions of these quantities were reported: one where branches smaller than 5 µm were excluded and another where branches smaller than 15 µm (2.5 µm and 7.5 µm for PND6) were excluded removing insignificant structures or artifacts. Some examples of the quantities reported from this analysis are the length of branches and the distance from a branch to the point of attachment, counted both in terms of the number of branching points and as the sum of the lengths of the branches that linked the two. Branching angles and the tortuosity of the branches (i.e., for a branch, the ratio between its length by the length of a straight line between its extremities: the less straight the branch, the higher the tortuosity) were also considered. Other quantities, such as the local thickness, both in 2D and 3D, were also determined by considering the average and standard deviation of their values on the skeleton points of every branch.
This automated quantitative method measures 139 structural features of mammary glands, 48 more than our previous work (see supplemental material in [19] ). These features represent morphological endpoints of physiological relevance, such as the complexity of the ductal tree, budding, epithelial area among others.
2.7. Statistical analysis of quantitative epithelial morphological features
2.7.1. The rationale for the statistical analysis
In this study design, there are 10–11 animals per treatment with three doses each of two chemicals as well as the control animals. Unlike more customary animal studies in which each individual animal yields a limited number of measurements for a small set of endpoints, this work generates measurements for 139 endpoints for the mammary gland of each individual rat. In this experimental design, there are circumstances that may interfere with a typical statistical analysis. First, in many cases, single features and specific doses will not be statistically significant alone because variability is naturally high due to the fact that animals are individuals [26] , and generic corrections of multiple comparisons cripple statistical significance. Second, the recurrence of a typical linear pattern could very well stem from a common, random origin since different features of an animal can be correlated [9] ; therefore, a more adequate statistical method for such analyses is required. To build on the diversity of features measured and to avoid errors stemming from multiple comparisons of non-independent variables, an analysis was designed at the level of all observed features combined with the relevant treatments (for example, the three doses of KETO). This strategy was tested and utilized in our previous work [19] and was recently reviewed [10] .
In this study, the different features observed for an animal are not independent a priori – for example, the total length of the epithelial branches and their volume are correlated. Also, when working with endocrine disrupting compounds, a linear response is not the only dose-response expected. The complex structure of this analysis necessitates the use of statistics more commonly associated with -omics datasets, such as those used in transcriptomics or metabolomics [6] . We have previously shown this statistical rationale to be appropriate for these types of automated measurements [19] . In the current study, we again use the Monte Carlo permutation test and the principal component analysis (PCA) for a more global analysis of the data. Unlike traditional tests, which use a standard distribution, the permutation test builds the statistic based on the data and a specific statistical hypothesis. Because DES is an estrogen and KETO affects the synthesis of estrogens, additional statistical tests are run to ascertain whether their effects in the mammary gland are similar.
2.7.2. Permutation test
Like any statistical test, the permutation test requires a null hypothesis and an alternative hypothesis that, in the observed data, a random variable (X) would reach a value that is too far from its expected mean under the null hypothesis to be probable, with a threshold of p = 0.05.
The Monte Carlo permutation test is performed using the null hypothesis that all experimental samples follow the same distribution. The first step is to define and compute the random variable (X) on the observed data. The second step is to pool all sample data and resample them, maintaining the group size for each treatment. This operation of permutation is equivalent to randomly assigning the condition (exposure) of each animal but holding the number of animals for every condition constant and, for each individual animal, preserving all its measured biological properties. Since all variables describing individual animals except their condition (exposure dose) are left unchanged, all correlations between the morphological features in data sets are preserved in the permutations and considered in the test. Moreover, under the null hypothesis that the treatment is inconsequential, this permutation of the treatment labels preserves the statistical properties of the data. The third step is to compute the value of X on the permuted data. Step four is to iterate steps two and three a given number of times (10,000 in our case). Step five is to regroup the results of step four to obtain the distribution of X under the null hypothesis, and then to assess whether X observed in the original data, computed in step 1, is in the 95 % confidence range around the mean of X. If X observed is outside this range, the null hypothesis is rejected in favor of the alternative hypothesis. This analysis has also been further described in Montévil et al. [19] .
2.7.2.i. Effect of treatment dose
Since the number of doses is limited, we could only assess linear responses. To this end we defined the random variable (X) as the number of properties (endpoints) for which there is a linear response among the three doses used with p < 0.05 by ANOVA. Then, the alternative hypothesis is that X is above the mean, that is to say, the data have more linear trends with respect to treatment doses than expected by chance alone ( Fig. 4 A).
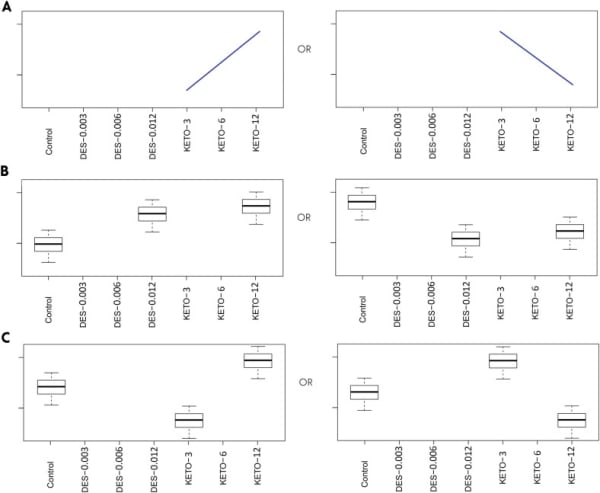
Fig. 4 . Patterns tested with the permutation test. A: linear trends among the three doses. B: consistency of the high dose effect of KETO and DES with respect to control. C: Low and high doses have opposite effects with respect to control.
2.7.2.ii. Consistency between KETO and DES effects
To expand on the above result, the effect of the highest dose of DES was assessed for consistency with the KETO trend. To this end, the permutation test was used in two different ways, that is, for two different random variables. The first counted the number of endpoints which show a linear trend for KETO and for which the difference between the mean of control and DES 0.012 and the mean of control and KETO 12 have the same sign i.e., same trend or direction, Fig. 4 B). Secondly, since the prevalence of linearity may complicate the interpretation of the above, the permutation test was then restricted to only those endpoints that previously displayed a linear response for KETO. In this second case, the random variable was defined as the number of endpoints for which the difference between the mean of control and DES 0.012 and the mean of control and KETO 12 have the same sign (i.e., same trend), and this difference has a threshold p-value lower than 0.5 by a t -test, to remove excessive noise ( Fig. 4 B).
2.7.2.iii. Assessment of non-monotonicity
The number of doses tested in this dataset is insufficient to directly ascertain the presence of non-monotonic dose responses. However, there is an indirect way to do this, namely by examining the effects of the doses with respect to the control value [35] . For example, if a low dose of KETO and a high dose of KETO have a consistent (same sign) or opposite effect (opposite sign) compared to the control. In the case of an opposite effect, the effect would be due to a non-monotonic relationship between dose and effect. To this end, we used the same random variables as in 2.7.2.ii, looking instead at the difference between the mean of control and KETO 3 and the mean of control and KETO 12 having opposite signs ( Fig. 4 C).
2.7.3. Linear responses
Because a lack of significance in the permutation test could be a consequence of large variability or of only a few variables displaying this trend, linearity was still assessed independently for each variable in case the negative results were false negatives. To analyze the linearity of individual properties, we used the analysis of variance implemented in R, a programming language for statistical computing and graphics supported by the R Core Team and the R Foundation for Statistical Computing [14] .
2.7.4. Principal component analysis
A principal component analysis (PCA) was performed using R and the FactoMineR package. The “dimdesc function” of this package identifies which variables are contributing to the differences in dimensions resulting from PCA and the effect of treatments; for further details, see Supplementary Analysis by PCA, Supplemental Material in [19] . Results are expressed as (variables, correlation coefficient, and p-value).
2.7.5. Mean comparisons
The Student’s t - test was used to perform comparisons between the control and the highest doses in PND22; since the permutation test singularizes them as having the largest effect. For other doses and in PND6, Dunnett’s test was used to accommodate multiple comparisons. In the box plots, outliers were defined using the 1.5 interquartile range method.
3. Results
The global permutation tests revealed significant results in the PND22 mammary glands. For this reason, we will report this later timepoint before the earlier PND6.
3.1. PND22 timepoint
At this time point, the overall trend assessed by the permutation test was different for the two treatment compounds. DES did not show any significant trend (p = 0.28), but KETO (p = 0.03) did. There is a significant linear response in 43 variables in the KETO treatment group, and the result is not due to correlations between features observed since the permutation test takes them into account.
The effect of the highest DES dose is consistent with the trend of the variables that have a linear response (p = 0.0085) and when restricting the analysis to the variables with a linear response (p = 0.032). That is, all but two variables among the 43 show a similar effect at the highest DES and KETO doses. Both of these variables are the angle with regard to the main direction of the branch but with two different size thresholds.
Finally, the lowest dose and the highest dose of KETO have opposite effects with respect to control (p = 0.018 and p = 0.005 when restricting the analysis to the 43 variables having a linear response). This result supports non-monotonicity.
Examples of variables with linear trends for KETO are: Feret diameter (p = 0.0015), Convex Surface Area (p = 0.0091), 2D Area (p = 0.015), Volume (p = 0.026) and Branches (p = 0.045) ( Fig. 5 ).
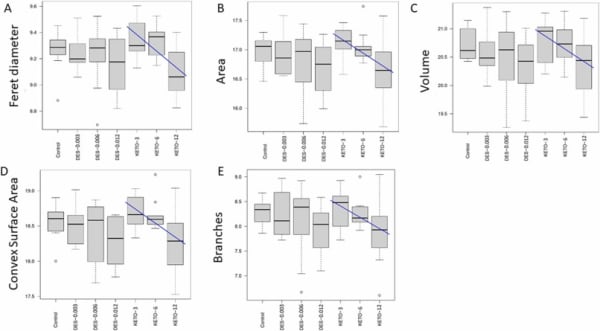
Fig. 5 . Panels A to E illustrate mean values of 5 of the 43 variables showing a significant linear trend for KETO. Note that in all cases there are dose dependent decreases in the KETO treatment and that the effect of the highest DES dose is similar to KETO. The blue line illustrates a monotonic dose response curve. Circles above bars indicate outlier values.
In the PND22 mammary glands, there were a large number of significant linear responses in the KETO treatment ( Table 1 ) that were not significant in the DES-treated animals. However, the DES-treated animals did have significant linear responses in 6 endpoints measured. Four of them did not have a linear response in KETO (minor axis, length of the smallest semi-axis, second radius of the ellipse, and bud volume), and two were linear in DES and KETO (minimum Feret diameter and oriented box width). These significant endpoints were associated with a reduction in size ( Table 1 ) and complexity of the epithelial structures in the DES ( Fig. 6 A) and KETO ( Fig. 6 B) treated glands. Of note, two dimensions identified in the PCA test were significant for KETO 6 while only a single dimension was significant for DES (Supplemental F1–2).
Table 1 . Morphometric endpoints collected from image analysis in PND 22 mammary glands, which were identified as having either an increasing trend (indicated by + and dark gray shading, p < 0.05) or a decreasing trend (indicated by – and light gray shading, p < 0.05) using the statistical test indicated at the top of the column.
(K3) indicates KETO 3 mg dose
(K6) indicates KETO 6 dose
(D3) indicates DES 0.003 dose
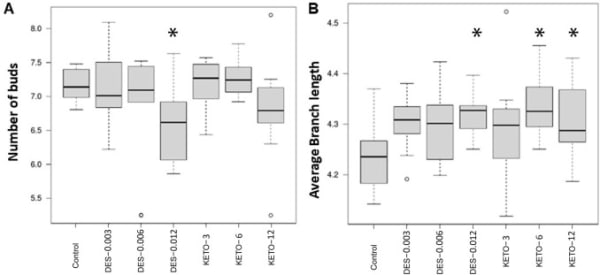
Fig. 6 . PND 22: Panel A: bud number; Panel B: average branch length; both measurements indicate a decrease of complexity of epithelial structures (* indicates p < 0.05 compared to controls. Circles above or below bars indicate outlier values).
There are significant differences in the number of buds and the average branch length between the control and the highest dose of DES, as indicated by the t -test. The average branch length is also significantly higher than the control for KETO6 and KETO12 values ( Fig. 6 ).
3.2. Early timepoint: PND6
In the PND6 animals, the mammary glands were not exposed to endogenous ovarian estrogens. One trend in this timepoint was that neither DES (p = 0.488) nor KETO (p = 0.552) were significant overall in the permutation test. In an independent test for linearity by ANOVA, there were significant responses for several of the 139 structural features ( Table 2 ).
Table 2 . Morphometric endpoints collected from image analysis in PND 6 mammary glands which were identified as having either an increasing trend (indicated by + and dark gray shading, p < 0.05) or a decreasing trend (indicated by – and light gray shading, p < 0.05) using the statistical test indicated at the top of the column.
(K3) indicates KETO 3 mg dose
(D3) indicates DES 0.003 dose
There were significant differences in several endpoints for the lowest doses of both DES and KETO with some of the endpoints overlapping ( Table 2 ). Additionally, when the linear trend by ANOVA is positive the lowest dose is generally significantly below the control. Examples of this are found in Table 2 : for DES, the average number of bifurcations, and for KETO, skewness. This explains why the lowest dose is the only one that differs from the control as the trend reverses within the response ( Fig. 7 ). Only a single dimension was significant in the PCA at KETO3 while none were in DES (Supplemental F3–4).
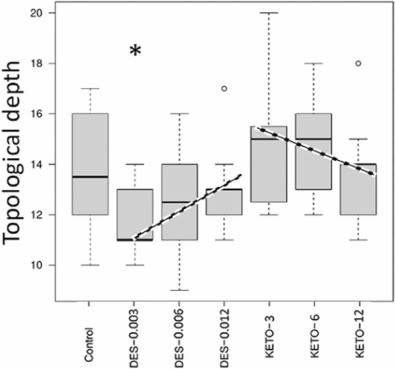
Fig. 7 . PND6: Topological depth. DES and KETO dose represent mg/kg bw/day. The circles above the bars represent outlier values. Dashed line indicates a non-significant trend. * Indicates p < 0.05 compared with control.
Statistically significant differences (p = 0.02 by ANOVA) in topological depth, defined as the number of bifurcations from the nipple to the farthest branch, were observed in DES-treated PND6 glands ( Fig. 7 ).
Normal mammary gland parenchymal development is characterized by increased size and complexity over time ( Table 3 ). The mammary glands of PND6 and PND22 animals showed significant differences among treatment groups at both ages. At PND6 differences between control and individual dose groups for DES and KETO were only significant for markers of complexity but not for size, and only at the lowest doses ( Table 2 ). At PND22 several variables indicate that the gland parenchymal size is decreased when the highest doses of DES and KETO are compared to controls (number of branches, epithelial area, epithelial volume, width and extension of the gland epithelium, illustrated in Fig. 5 ). In addition, the ductal system is less complex (decreased bud number, increased average branch length, illustrated in Fig. 6 ). In contrast, the commonly used subtended area measurement and the TEB numbers were not affected by treatment (not shown).
Table 3. Mean control values (+/- SD) of 5 commonly assessed mammary gland endpoints at PND6 and PND22.
Empty Cell | Area (2d) (mm 2 ) | Volume (mm 3 ) | Number of branches | Average Branch Length (µm) | Number of buds |
---|---|---|---|---|---|
PND6 | 5.2 ± 2.4 | 0.11 ± 0.054 | 2.9 × 10 3 ± 1.5 × 10 3 | 39 ± 4.7 | 9.8 × 10 2 ± 3.8 × 10 2 |
PND22 | 24.0 ± 5.7 | 1.02 ± 0.290 | 4.1 × 10 3 ± 9.9 × 10 2 | 69 ± 5.3 | 1.3 × 10 3 ± 3.2 × 10 2 |
4. Discussion
The objectives of this study were to assess the effect of the endocrine disruptors KETO and DES in the developing mammary gland and to compare the sensitivity of the rat mammary gland with traditional reproductive endpoints measured in the same set of animals at the two time points (PND6 and PND22). Mammary development is studied using the quantitative automatic methodology we recently developed [19] and refined. Consistent with clinical data showing gynecomastia in 20 % of men treated with KETO [3] and other triazide fungicides like posaconazole [33] , we observed that this fungicide affects mammary gland development.
PND22 showed a much larger number of significant endpoints affected than PND6. This difference is consistent with the interpretation that the initial effect of KETO and DES is magnified by exposure to endogenous ovarian estrogens, which start to be secreted around PND12 [25] . However, although the number of significant parameters is lower in PND6 than in PND22, the differences at PND6 manifest at the lowest dose tested for both DES and KETO, suggesting that the rat mammary gland is more sensitive at a younger age. The scarcity of significant endpoints at the lowest dose in PND22 could possibly be due to the presence of endogenous estrogens at this age which might decrease the differences between controls and treated animals.
One challenge when using a 2D projection of the image of the mammary gland results from the structure of the ductal tree, which is growing in the three dimensions of space. This fact limits the analysis of the rat mammary gland to ages equal to or younger than PND22 using confocal microscopy. Indeed, the thickness of the MG at PND6 and PND22 allowed for the collection of confocal images and a complete 3D reconstruction, although this process did prove time-consuming. This, in turn, allowed for the precise measurements which constitute the major contribution of this work. In contrast, the increased thickness of the gland after PND22 precludes the inclusion of later time points in this precise analysis.
A linear dose-response curve to KETO (the 3 doses excluding the control, ANOVA p < 0.05) was found in 43 variables. The probability that at least 43 variables would randomly display this pattern was negated by the permutation test result of a p = 0.028 thus suggesting a strong likelihood that the effect is due to the treatment. The current study design with only 3 doses per treatment did not allow for the direct assessment of non-monotonicity, the original motivation for the development of this methodology, since the semi-quantitative ones are not as well suited for this purpose, particularly when the specimens are evaluated in reference to both a positive and negative control [19] . This, however, could be corrected by including high- and low- dose positive controls.
As stated above, several of these variables indicate that the gland size and complexity are decreased by increased KETO doses at the later timepoint; some measurements are significant at the 2 highest doses of KETO. The highest dose of DES also had an effect comparable to that of the highest dose of KETO. The relevant issue is not the magnitude of the effect, but its direction, i.e., if KETO decreases the variable, so does DES, as indicated in Fig. 5 . This phenomenon holds true for 41 of the 43 variables with p = 0.032 by a permutation test. This result corroborates the trend observed with KETO in different animals. The average branch length increased; this means that the branches bifurcate at a lower frequency than in the control; this applies both to DES and KETO. Additionally, at the highest doses tested, both DES and KETO exposure decreased bud number; this is consistent with decreased formation of branches and alveolar structures. Although the 3 doses of DES and of KETO showed directionality as expected in a monotonic dose response curve, a nonmonotonic dose response curve cannot be ruled out as only the control and 3 doses of each chemical were tested. Regarding KETO, the lowest dose tended to have the opposite effect as that of the highest dose when compared to the control ( Fig. 5 C), suggesting a non-monotonic dose response curve. Of note, all tested doses yielded some significant effects in different endpoints.
The MG samples analyzed here were taken from the animals in the study reported in [11] . The latter paper showed that classical toxicological female reproductive endpoints were insensitive to DES and KETO [11] ; the highest DES dose caused slightly longer anogenital distance, while the 3 DES doses showed a slight delay in the vaginal opening, as did the intermediate KETO dose. Another part of the study investigated the effects of DES and KETO on the hypothalamic control of puberty in female rats. The results showed a delay in the maturation of gonadotropin-releasing hormone (GnRH) secretion before puberty by a decreased pulsatility of GnRH secretion, a result consistent with delays in the vaginal opening. Transcriptome analysis revealed that a high number of genes regulating the activity of the extrinsic GnRH pulse generator were consistently affected by all the doses of DES and KETO before puberty [5] . The study on hypothalamic control of puberty suggested potential biomarkers for assessing the effects of such chemicals on reproductive health. These could, together with the assessment of mammary glands, be included in future testing strategies for EDC identification.
Several classical female reproductive endpoints were not affected at the tested doses of DES or KETO. Both the in vitro study of GnRH secretion and the morphometric analysis described herein showed significant differences between the control and the highest doses of DES or KETO. However, in the mammary gland, significant effects were also observed at the intermediate and lowest doses of KETO at PND22 and at the lowest dose of KETO and DES at PND6. This is similar to the transcriptomic analysis of hypothalamic tissues, which revealed alterations at all doses tested [5] .
5. Conclusions
These results show that, as inferred, ketoconazole affects the development of the mammary gland parenchyma. The mammary gland is sensitive to the doses of DES and KETO tested in this experiment, with several variables indicating that the gland parenchyma size is decreased, and the ductal system is less complex. This agrees with the findings of delayed pubertal onset and delay in GnRH pulsatility in animals from the same study. The consistent, gradual decline in measurements across the three KETO doses indicates a clear directionality, highlighting the robustness of the endpoint being affected at two out of the three doses tested.
These results support the potential inclusion of the PND22 (weanling) mammary gland whole mounts in future international test methods for reproductive toxicity.
While advanced mammary morphology studies using 3D scans are unlikely to become standard requirements in regulatory toxicology studies in the near future, our results demonstrate the potential for identifying key endpoints. These findings suggest that further exploration and improvement of simpler quantitative image analysis methods could be beneficial.
Moreover, the permutation test is a versatile and straightforward tool that may be used to assess specific trends in complex data, such as dose responses, even when there are fewer endpoints than in this study.
Funding
This work was funded by the EU Horizon 2020 project FREIA [grant number 825100 ] van Duursen et al. [4]
Credit authorship contribution statement
Christiansen Sofie: Writing – review & editing, Project administration, Methodology. Boberg Julie: Writing – review & editing, Project administration, Methodology, Funding acquisition. Schaeberle Cheryl: Writing – review & editing, Writing – original draft, Methodology. Montévil Maël: Writing – review & editing, Writing – original draft, Validation, Software, Investigation, Formal analysis, Conceptualization. Soto Ana: Writing – review & editing, Writing – original draft, Methodology, Funding acquisition, Conceptualization.
Declaration of Competing Interest
The authors declare the following financial interests/personal relationships which may be considered as potential competing interests: Ana M. Soto reports financial support was provided by EU Horizon 2020. AMS has received travel re-imbursements from Universities, Governments and NGOs to speak about endocrine disruption, plastics and environmental causes of cancer. The other authors declare that they have no known competing financial interests or personal relationships that could have appeared to influence the work reported in this paper
Acknowledgements
We would like to thank laboratory technicians Mette Voigt Jessen, Dorte Lykkegaard Korsbech, Lillian Sztuk, and Heidi Broksø Letting for excellent work with the mammary glands, as well as the personnel in the Bio Facility at DTU for their work during the animal studies. We also thank Victoria Bouffard in the Soto laboratory for her critical reading of the manuscript.
Appendix A. Supplementary material
Supplementary material
References
- [1] N. Acevedo, B. Davis, C.M. Schaeberle, C. Sonnenschein, A.M. Soto Perinatally administered Bisphenol A as a potential mammary gland carcinogen in rats Environ. Health Perspect., 121 (2013), pp. 1040-1046
- [2] H.A. Bern, M. Edery, K.T. Mills, A.F. Kohrman, T. Mori, L. Larson Long- term alterations in histology and steroid receptor levels of the genital tract and mammary gland following neonatal exposure of female BALB/cCrgl mice to various doses of diethylstilbestrol Cancer Res., 47 (1987), pp. 4165-4172
- [3] F. Deepinder, G.D. Braunstein Drug-induced gynecomastia: an evidence-based review Expert Opin. Drug Saf., 11 (5) (2012), pp. 779-795
- [4] M. Duursen, J. Boberg, S. Christiansen, L. Connolly, P. Damdimopoulou, P. Filis, P.A. Fowler, B.M. Gadella, J. Holte, K. Jääger, H.K.L. Johansson, T. Li, S. Mazaud-Guittot, A.S. Parent, A. Salumets, A.M. Soto, T. Svingen, A. Velthut-Meikas, E.B. Wedebye, Y. Xie, M.V.D. Berg Safeguarding Female Reproductive Health against Endocrine Disrupting Chemicals-The FREIA Project." Int J. Mol. Sci., 21 (9) (2020) 10.3390
- [5] D. Franssen, H.K.L. Johansson, D. Lopez-Rodriguez, A. Lavergne, Q. Terwagne, J. Boberg, S. Christiansen, T. Svingen, A.S. Parent Perinatal exposure to the fungicide ketoconazole alters hypothalamic control of puberty in female rats Front Endocrinol. (Lausanne), 14 (2023), p. 1140886
- [6] W.W.B. Goh, L. Wong Dealing with confounders in omics analysis Trends Biotechnol., 36 (5) (2018), pp. 488-498
- [7] N. Hasan, C. Sonnenschein, A.M. Soto Vitamin D3 constrains estrogen's effects and influences mammary epithelial organization in 3D cultures Sci. Rep., 9 (1) (2019), p. 7423
- [8] N. Hasan, Y. Zhang, I. Georgakoudi, C. Sonnenschein, A.M. Soto Matrix composition modulates vitamin D3's effects on 3D collagen fiber organization by MCF10A cells Tissue Eng. Part A, 27 (21-22) (2021), pp. 1399-1410
- [9] J.J. Heindel, S. Belcher, J.A. Flaws, G.S. Prins, S.M. Ho, J. Mao, H.B. Patisaul, W. Ricke, C.S. Rosenfeld, A.M. Soto, F.S. Vom Saal, R.T. Zoeller Data integration, analysis, and interpretation of eight academic CLARITY-BPA studies Reprod. Toxicol., 98 (2020), pp. 29-60
- [10] K.L. Howdeshell, B.E.J. Beverly, R.B. Blain, A.E. Goldstone, P.A. Hartman, C.R. Lemeris, R.R. Newbold, A.A. Rooney, J.R. Bucher Evaluating endocrine disrupting chemicals: A perspective on the novel assessments in CLARITY-BPA Birth Defects Res, 115 (15) (2023), pp. 1345-1397
- [11] H.K.L. Johansson, S. Christiansen, M.K. Draskau, T. Svingen, J. Boberg Classical toxicity endpoints in female rats are insensitive to the human endocrine disruptors diethylstilbestrol and ketoconazole Reprod. Toxicol., 101 (2021), pp. 9-17
- [12] J.E. Kay, B. Cardona, R.A. Rudel, L.N. Vandenberg, A.M. Soto, S. Christiansen, L.S. Birnbaum, S.E. Fenton Chemical effects on breast development, function, and cancer risk: existing knowledge and new opportunities Curr. Environ. Health Rep., 9 (4) (2022), pp. 535-562
- [13] M.B. Kjærstad, C. Taxvig, C. Nellemann, A.M. Vinggaard, H.R. Andersen Endocrine disrupting effects in vitro of conazole antifungals used as pesticides and pharmaceuticals Reprod. Toxicol., 30 (4) (2010), pp. 573-582
- [14] S. Lê, J. Josse, F. Husson FactoMineR: an R package for multivariate analysis J.Stat. Softw., 25 (1) (2008), pp. 1-18
- [15] D. Legland, I. Arganda-Carreras, P. Andrey "MorphoLibJ: integrated library and plugins for mathematical morphology with ImageJ Bioinformatics, 32 (22) (2016), pp. 3532-3534
- [16] K.R. Mandrup, H.K. Johansson, J. Boberg, A.S. Pedersen, M.S. Mortensen, J.S. Jørgensen, A.M. Vinggaard, U. Hass Mixtures of environmentally relevant endocrine disrupting chemicals affect mammary gland development in female and male rats Reprod. Toxicol., 54 (2015), pp. 47-57
- [17] C.M. Markey, E.H. Luque, M.M. Munoz de Toro, C. Sonnenschein, A.M. Soto In utero exposure to bisphenol A alters the development and tissue organization of the mouse mammary gland Biol. Reprod., 65 (2001), pp. 1215-1223
- [18] K. Matouskova, G.K. Szabo, J. Daum, S.E. Fenton, S. Christiansen, A.M. Soto, J.E. Kay, B. Cardona, L.N. Vandenberg Best practices to quantify the impact of reproductive toxicants on development, function, and diseases of the rodent mammary gland Reprod. Toxicol. (2022)
- [19] M. Montévil, N. Acevedo, C.M. Schaeberle, M. Bharadwaj, S.E. Fenton, A.M. Soto A combined morphometric and statistical approach to assess nonmonotonicity in the developing mammary gland of rats in the CLARITY-BPA study Environ. Health Perspect., 128 (5) (2020), p. 57001
- [20] C.H. Munkboel, T.B. Rasmussen, C. Elgaard, M.K. Olesen, A.C. Kretschmann, B. Styrishave The classic azole antifungal drugs are highly potent endocrine disruptors in vitro inhibiting steroidogenic CYP enzymes at concentrations lower than therapeutic Cmax Toxicology, 425 (2019), Article 152247
- [21] T.J. Murray, M.V. Maffini, A.A. Ucci, C. Sonnenschein, A.M. Soto Induction of mammary gland ductal hyperplasias and carcinoma in situ following fetal Bisphenol A exposure Reprod. Toxicol., 23 (2007), pp. 383-390
- [22] R. Nicotra, C. Lutz, H.A. Messal, J. Jonkers Rat models of hormone receptor-positive breast cancer J. Mammary Gland Biol. Neoplasia, 29 (1) (2024), p. 12
- [23] T. Paulose, L. Speroni, C. Sonnenschein, A.M. Soto Estrogens in the wrong place at the wrong time: Fetal BPA exposure and mammary cancer Reprod. Toxicol., 54 (2015), pp. 58-65
- [24] A.A. Peters, W.V. Ingman, W.D. Tilley, L.M. Butler Differential effects of exogenous androgen and an androgen receptor antagonist in the peri- and postpubertal murine mammary gland Endocrinology, 152 (10) (2011), pp. 3728-3737
- [25] C.A. Picut, D. Dixon, M.L. Simons, D.G. Stump, G.A. Parker, A.K. Remick Postnatal ovary development in the rat: morphologic study and correlation of morphology to neuroendocrine parameters Toxicol. Pathol., 43 (3) (2015), pp. 343-353
- [26] B.S. Rubin, C.M. Schaeberle, A.M. Soto The case for BPA as an obesogen: contributors to the controversy Front Endocrinol. (Lausanne), 10 (2019), p. 30
- [27] T. Satoh, H. Munakata, K. Fujita, S. Itoh, S. Itoh, T. Kamataki, I. Yoshizawa Studies on the interactions between drug and estrogen. II. On the inhibitory effect of 29 drugs reported to induce gynecomastia on the oxidation of estradiol at C-2 or C-17 Biol. Pharm. Bull., 26 (5) (2003), pp. 695-700
- [28] T. Satoh, Y. Tomikawa, K. Takanashi, S. Itoh, S. Itoh, I. Yoshizawa Studies on the interactions between drugs and estrogen. III. Inhibitory effects of 29 drugs reported to induce gynecomastia on the glucuronidation of estradiol Biol. Pharm. Bull., 27 (11) (2004), pp. 1844-1849
- [29] K.G. Sheets, J. Bokkyoo, Y. Zhou, J. Winkler, N. Petasis, W.C. Gordon, N.G. Bazan Topical neuroprotectin D1 attenuates experimental CNV and induces activated microglia redistribution Invest Opthalmol Vis. Sci. ARVO Meet. Abstr., 52 (2011), p. 5470
- [30] A.M. Soto, C. Brisken, C.M. Schaeberle, C. Sonnenschein Does cancer start in the womb? Altered mammary gland development and predisposition to breast cancer due to in utero exposure to endocrine disruptors J. Mammary Gland Biol. Neoplasia, 18 (2013), pp. 199-208
- [31] A.M. Soto, C. Sonnenschein, K.L. Chung, M.F. Fernandez, N. Olea, M.F. Olea-Serrano The E-SCREEN assay as a tool to identify estrogens: an update on estrogenic environmental pollutants Environ. Health Perspect., 103 (1995), pp. 113-122
- [32] L. Speroni, M. Voutilainen, M.L. Mikkola, S.A. Klager, C.M. Schaeberle, C. Sonnenschein, A.M. Soto New insights into fetal mammary gland morphogenesis: differential effects of natural and environmental estrogens Sci. Rep., 7 (2017), p. 40806
- [33] G.R. Thompson, 3rd, P.N. Surampudi, A. Odermatt Gynecomastia and hypertension in a patient treated with posaconazole Clin. Case Rep., 8 (12) (2020), pp. 3158-3161
- [34] L.N. Vandenberg, M.V. Maffini, P.R. Wadia, C. Sonnenschein, B.S. Rubin, A.M. Soto Exposure to environmentally relevant doses of the xenoestrogen bisphenol-A alters development of the fetal mouse mammary gland Endocrinology, 148 (2007), pp. 116-127
- [35] L.N. Vandenberg, P.R. Wadia, C.M. Schaeberle, B.S. Rubin, C. Sonnenschein, A.M. Soto The mammary gland response to estradiol: monotonic at the cellular level, non-monotonic at the tissue-level of organization?" J. Steroid Biochem. Mol. Biol., 101 (2006), pp. 263-274
- [36] P.R. Wadia, N.J. Cabaton, M.D. Borrero, B.S. Rubin, C. Sonnenschein, T. Shioda, A.M. Soto Low-dose BPA exposure alters the mesenchymal and epithelial transcriptomes of the mouse fetal mammary gland PLoS One, 8 (2013), Article e63902
- [37] G. Zinser, K. Packman, J. Welsh Vitamin D(3) receptor ablation alters mammary gland morphogenesis Development, 129 (13) (2002), pp. 3067-3076